Clinical scale patient reported outcomes are commonly used research tools that can be designed to provide quantitative or qualitative outputs. These assessments use objective scales and/or qualitative, open-ended responses that rely on self-reporting to collect objective data and descriptions of thoughts, feelings, and behaviors, making assessment methodologies particularly attractive in psychiatric and psychological research. These approaches allow researchers to gather a large quantity of data, relatively quickly, and provide a representative sample population being investigated, acknowledging and controlling for the risk of responder bias.1
The Challenge of the Placebo Response in Clinical Trials for Psychiatric Disorders
The placebo response is a well-known confounding factor in clinical trials.2 This is a phenomenon in which a patient’s symptoms improve due to their perception or belief in the treatment as opposed to treatment effect. In turn, the placebo effect can lead to increased heterogeneity in clinical trial results, diminishing study power and impairing measurement of treatment efficacy.
Clinical trials for psychiatric disorders, such as bipolar disorder, tend to have high placebo response rates, resulting in the need to increase sample size to adequately power analyses of efficacy.3 In studies of acute mania, placebo response rates are 31% on average, and studies of acute bipolar depression carry an average of 39% placebo response rates.4
Several factors, including patient expectations for improvement, therapeutic setting, extra care, and interaction with healthcare providers in the research setting, the use of adjunctive behavioral and pharmacologic therapies, and the natural history or course of illness all contribute to the placebo response. Younger individuals, a higher percentage of males, and the presence of psychotic symptoms are predictors of increased drug-placebo differences and a decreased placebo response in bipolar disorder studies.
In attempts to minimize the placebo response and increase drug-placebo differences, several study controls have been suggested, including: maximizing the cohort size of patients randomized to placebo, decreasing or controlling adjunctive supportive care during the trial, improving study blinding, and requiring longer durations of illness before starting the trial have been suggested.
The Role of Machine Learning and Artificial Intelligence in Elucidating the Placebo Response
Artificial intelligence (AI) can help mitigate the impact of the placebo response in clinical trials in several ways. Techniques including Bayesian optimization, decision trees, random forests, and neural networks can be used to optimize clinical trial design. These techniques can help identify the optimal patient population, treatment regimen, and outcome measures for a given trial. They can also be used to identify subgroups of patients who are most likely to respond to a treatment, and to identify subgroups of patients who may be more or less susceptible to the placebo effect.5
Unfortunately, attempts to elucidate the placebo response, and understand why some patients respond to placebo using traditional ML methods have largely failed. Common clustering algorithms are incapable of teasing out subtleties in data due to the supplemental complexity of unquantified or unconsidered factors such as patient actions and expectations.
NetraMark’s Work with Clinical Scale Data on the Placebo Response
NetraMark utilized clinical scale data from populations with psychiatric diagnoses to derive insights into the heterogeneity inherent to these datasets. A number of assessments, broadly classified into clinical scales, have been developed by psychiatrists to provide continuous and categorical data outputs that NetraAI can analyze. Using clinical scale data, NetraAI was able to accurately model placebo responses from these datasets.
Using NetraAI to Predict Placebo Response for Clinical Trials in Bipolar Disorder
NetraAI created a model for Placebo Non-Response. The data used was from a failed drug trial meant to evaluate depression in Bipolar Disorder Type 1 from Takeda (NCT01467700). It consisted of 64 placebo patients and 150 variables using clinical data and clinical rating scale data including the Hamilton Rating Scale for Depression (HAM-D), Montgomery-Asberg Depression Rating Scale (MADRS)6, and the Placebo Response Probability Score (PRPS).7
Integrating Scale and Assessment Data to Understand Patient Populations in Clinical Trials
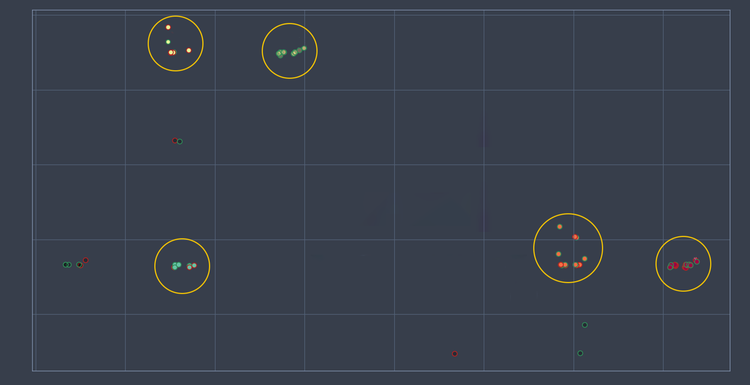
Figure 1. A mosaic of patient subpopulations characterized by different sets of questions from a set of scale questions.
NetraAI was utilized to integrate several clinical scales such as the HAM-D, Hamilton Rating Scale for Anxiety (HAM-A), Young Mania Rating Scale (YMRS), NEO Personality Inventory, and other scales. The goal was to understand if a patient population could be segmented into subpopulations that could explain response to both drug and to placebo by using self-reported and clinic collected scale data. Sponsors utilize considerable resources to collect these data and thus the NetraAI becomes a powerful tool, in conjunction with the scales, to inform and enrich phase III trials from phase II data. Further, these data identify subpopulations of patients with medically unmet needs, which allows pharmaceutical companies to refine future trials, while avoiding failure in current clinical trials.
As an example, in this client study NetraAI was able to integrate several scales and create a mosaic of patient subpopulations that were characterized by a set of questions derived from several assessment instruments (Figure 1). This is an example of a patient stratification made possible due to assessment data.
In this example, there was a collection of questions that were discovered to characterize the drug response in a set of patients. These questions came from clinical scales HAM-A, MADRS, Quick Inventory of Depressive Symptomatology (QIDS), YMRS, and others (Table 1). This collection of questions are related to mental health assessments or evaluations focused on symptoms of anxiety and depression. Considering the overlapping themes in the questions, they may present a set of exclusion criteria that can be used for sorting patient inclusion in future trials.
Table 1. Variables characterizing drug response.
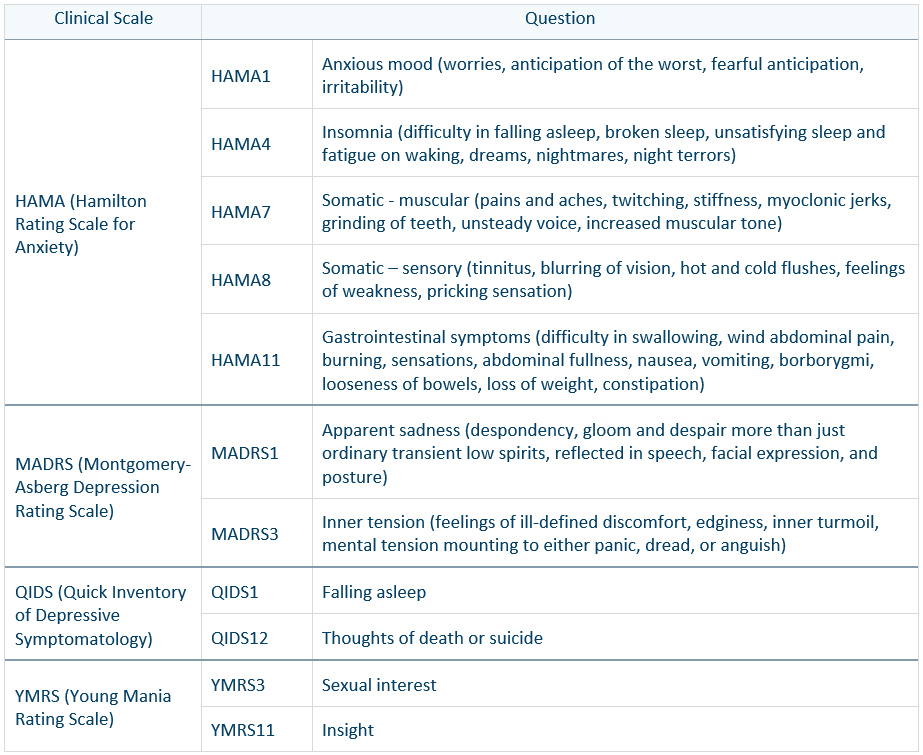
Significance of Using NetraAI with Assesment Data to Generate Subgroup Hypotheses for Phase III Patient Enrichment
The NetraAI’s ability to generate hypotheses about patient populations allows it to help pharmaceutical companies by enriching their Phase III trials through insights gained from Phase II data. We have shown from previous studies how molecular data, such as gene expression and methylation, can provide insights about drug response but collecting these data can be expensive. Sponsors can utilize scales and patient diaries to transform a complex patient population into a mosaic of well-defined subpopulations. This has the potential to reduce costs and improve success rates for clinical trials.
References
-
- 7.1 Overview of Survey Research – Research Methods in Psychology. https://opentext.wsu.edu/carriecuttler/chapter/7-1-overview-of-survey-research/.
- Hafliðadóttir, S. H. et al. Placebo response and effect in randomized clinical trials: meta-research with focus on contextual effects. Trials 22, (2021).
- Cao, B. et al. Differential power of placebo across major psychiatric disorders: a preliminary meta-analysis and machine learning study. Scientific Reports 2021 11:1 11, 1–9 (2021).
- Rutherford, B. R., Roose, S. P. & Tandler, J. M. Placebo Response in Clinical Trials of Bipolar Disorder: Potential Causes, Potential Solutions. The Bipolar Book 585–598 (2015) doi:10.1093/MED/9780199300532.003.0041.
- Smith, E. A. et al. Using Artificial Intelligence-based Methods to Address the Placebo Response in Clinical Trials. Innov Clin Neurosci 19, 60 (2022).
- Tsay, Mi., Geraci, J. & Agrawal, A. Next-Gen AI for Disease Definition, Patient Stratification, and Placebo Effect. doi:10.31219/OSF.IO/PC7AK.
- Morlock, R. & Geraci, J. Placebo Response Propensity Scale (PRPS) Scores and Probability of Placebo Response by Disease Severity.